Data center management software is evolving — at last
Despite their role as enablers of technological progress, data center operators have been slow to take advantage of developments in software, connectivity and sensor technologies that can help optimize and automate the running of critical infrastructure.
Most data center owners and operators currently use building management systems (BMS) and / or data center infrastructure management (DCIM) software as their primary interfaces for facility operations. These tools have important roles to play in data center operations but have limited analytics and automation capabilities, and they often do little to improve facility efficiency.
To handle the increasing complexity and scale of modern data centers — and to optimize for efficiency — operations teams need new software tools that can generate value from the data produced by the facilities equipment.
For more than a decade, DCIM software has been positioned as the main platform for organizing and presenting actionable data — but its role has been largely passive. Now, a new school of thought on data center management software is emerging, proposed by data scientists and statisticians. From their point of view, a data center is not a collection of physical components but a complex system of data patterns.
Seen in this way, every device has a known optimal state, and the overall system can be balanced so that as many devices as possible are working in this state. Any deviation would then indicate a fault with equipment, sensors or data.
This approach has been used to identify defective chiller valves, imbalanced computer room air conditioning (CRAC) fans, inefficient uninterruptible power supply (UPS) units and opportunities to lower floor pressure — all discovered in data, without anyone having to visit the facility. The overall system — the entire data center — can also be modelled in this way, so that it can be continuously optimized.
All about the data
Data centers are full of sensors. They can be found inside CRAC and computer room air handler units, chillers, coolant distribution units, UPS systems, power distribution units, generators and switchgear, and many operators install optional temperature, humidity and pressure sensors in their data halls.
These sensors can serve as a source of valuable operational insight — yet the data they produce is rarely analyzed. In most cases, applications of this information are limited to real-time monitoring and basic forecasting.
In recent years, major mechanical and electrical equipment suppliers have added network connectivity to their products as they look to harvest sensor data to understand where and how their equipment is used. This has benefits for both sides: customers can monitor their facilities from any device, anywhere, while suppliers have access to information that can be used in quality control, condition-based or predictive maintenance, and new product design.
The greatest benefits of this trend are yet to be harnessed. Aggregated sensor data can be used to train artificial intelligence (AI) models with a view to automating an increasing number of data center tasks. Data center owners and operators do not have to rely on equipment vendors to deliver this kind of innovation. They can tap into the same equipment data, which can be accessed using industry-standard protocols like SNMP or Modbus.
When combining sensor data with an emerging category of data center optimization tools — many of which rely on machine learning — data center operators can improve their infrastructure efficiency, achieve higher degrees of automation and lower the risk of human error.
The past few years have also spawned new platforms that simplify data manipulation and analysis. These enable larger organizations to develop their own applications that leverage equipment data — including their own machine learning models.
The new wave
Dynamic cooling optimization is the best-understood example of this new, data-centric approach to facilities management. These applications rely on sensor data and machine learning to determine and continually “learn” the relationships between variables such as rack temperatures, cooling equipment settings and overall cooling capacity. The software can then tweak the cooling equipment performance based on minute changes in temperature, enabling the facility to respond to the needs of the IT in near real-time.
Many companies working in this field have close ties to the research community. AI-powered cooling optimization vendor TycheTools was founded by a team from the Technical University of Madrid. The Dutch startup Coolgradient was co-founded by a data scientist and collaborates with several universities. US-based Phaidra has brought together some of the talent that previously published and commercialized cutting-edge research as part of Google’s DeepMind.
Some of the benefits offered by data-centric management software include:
- Improved facility efficiency: through the automated configuration of power and cooling equipment as well as the identification of inefficient or faulty hardware.
- Better maintenance: by enabling predictive or condition-based maintenance strategies that consider the state of individual hardware components.
- Discovery of stranded capacity: through the thorough analysis of all data center metrics, not just high-level indicators.
- Elimination of human error: through either a higher degree of automation or automatically generated recommendations for human employees.
- Improvements in skill management: by analyzing the skills of the most experienced staff and codifying them in software.
Not all machine learning models require extensive compute resources, rich datasets and long training times. In fact, many of the models used in data centers today are small and relatively simple. Both training and inference can run on general-purpose servers, and it is not always necessary to aggregate data from multiple sites — a model trained locally on a single facility’s data will often be sufficient to deliver the expected results.
New tools bring new challenges
The adoption of data-centric tools for infrastructure management will require owners and operators to recognize the importance of data quality. They will not be able to trust the output of machine learning models if they cannot trust their data — and that means additional work on standardizing and cleaning their operational data stores.
In some cases, data center operators will have to hire analysts and data scientists to work alongside the facilities and IT teams.
Data harvesting at scale will invariably require more networking inside the data center — some of it wireless — and this presents a potentially wider attack surface for cybercriminals. As such, cybersecurity will be an important consideration for any operational AI deployment and a key risk that will need to be continuously managed.
Evolution is inevitable
Uptime Institute has long argued that data center management tools need to evolve toward greater autonomy. The data center maturity model (see Table 1) was first proposed in 2019 and applied specifically to DCIM. Four years later, there is finally the beginning of a shift toward Level 4 and Level 5 autonomy, albeit with a caveat — DCIM software alone will likely never evolve these capabilities. Instead, it will need to be combined with a new generation of data-centric tools.
Table 1. The Data Center Management Maturity Model
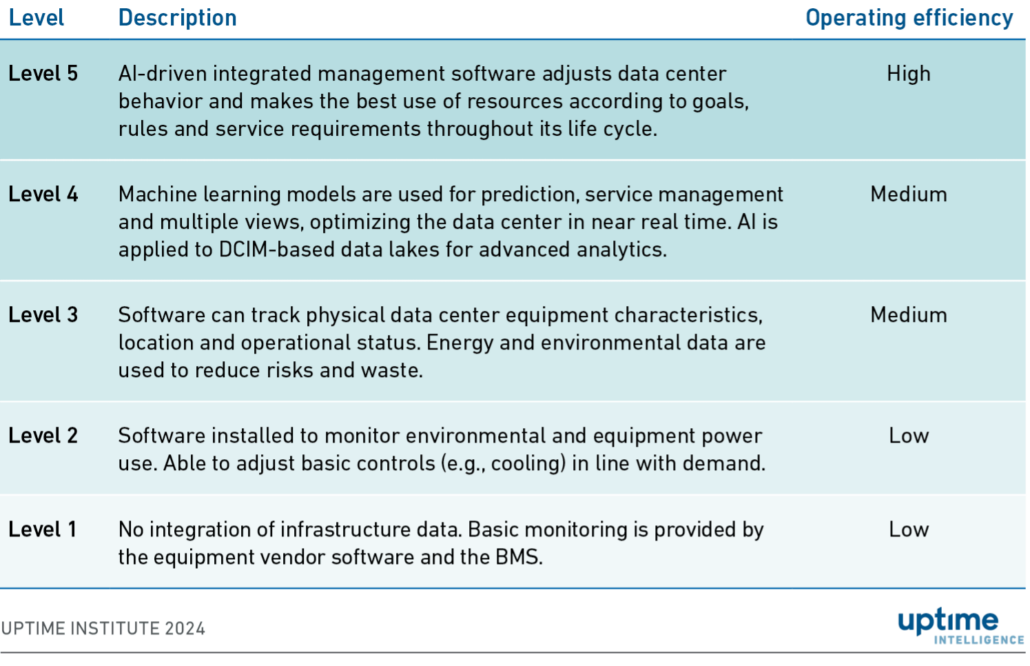
Not every organization will, or should, take advantage of Level 4 and Level 5 functionality. These tools will provide an advantage to the operators of modern facilities that have exhausted the list of traditional efficiency measures, such as those achieving PUE values of less than 1.3.
For the rest, being an early adopter will not justify the expense. There are cheaper and easier ways to improve facility efficiency that do not require extensive data standardization efforts or additional skills in data science.
At present, AI and analytics innovation in data center management appears to be driven by startups rather than established software vendors. Few BMS and DCIM developers have integrated machine learning into their core products, and while some companies have features in development, these will take time to reach the market — if they ever leave the lab.
Uptime Intelligence is tracking six early-stage or private companies that use facilities data to create machine learning models and already have products or services on the market. It is likely more will emerge in the coming months and years.
These businesses are creating a new category of software and services that will require new types of interactions with all the moving parts inside the data center, as well as new commercial strategies and new methods of measuring the return on investment. Not all of them will be successful.
The speed of mainstream adoption will depend on how easy these tools will be to implement. Eventually, the industry will arrive at a specific set of processes and policies that focus on benefitting from equipment data.
The Uptime Intelligence View
The design and capabilities of facilities equipment have changed considerably over the past 10 years, and traditional data center management tools have not kept up. A new generation of software from less-established vendors now offers an opportunity to shift the focus from physical infrastructure to data. This introduces new risks — but the benefits are too great to ignore.