AI will have a limited role in data centers — for now
The topic of artificial intelligence (AI) has captured the public’s imaginations, and now barely a week goes by without reports of another breakthrough. Among the many, sometimes dramatic predictions made by experts and non-experts alike is the potential elimination of some, or even many, jobs.
These expectations are partly — but only partly — mirrored in the data center industry: a quarter of respondents to the 2023 Uptime Institute annual data center survey believe that AI will reduce their data center operations staffing levels within the next five years. A much larger group, however, are more cautious, with nearly half believing that jobs will only be displaced over a longer period of time.
These views are inevitably speculative, but a measure of skepticism in the sector is understandable. Despite the hype surrounding large language models, such as ChatGPT, and other generative AI applications, the use cases for these AI tools in data center operations currently appear limited. There are, however, other forms of AI that are already in use in the data center — and have proved valuable — but have not affected any jobs.
AI-based technologies have been the subject of several hype cycles in the past, with their immediate impact always smaller than predicted. This supports the view that the transition to a new generation of software tools is unlikely to be quick, or as far-reaching in the near term, as some AI enthusiasts think.
There are two factors that will likely slow the impact of AI on data center jobs:
- The risk profile of most AI-based technologies is currently unacceptable to data center operators.
- Those AI-based technologies that have made their way into the data center appear to augment, rather than replace employees.
AI in context
AI is an inconveniently broad umbrella term used to describe computer software that is capable of exhibiting what humans perceive as intelligent behavior. The term includes disciplines such as machine learning (ML), which is concerned with developing complex mathematical models that can learn from data to improve model performance over time.
ML is important in the automation of certain tasks. Once trained on data center operational data, such models can react to events much faster and with more granularity than human employees. This attribute is the foundation for most of the current-generation AI-based data center applications, such as dynamic cooling optimization and equipment health monitoring.
But the term AI also includes plenty of other concepts that span a wide range of applications. The most hotly pursued approaches fashion deep neural networks into complex logic using a training process. Such systems address computational problems that cannot be explicitly expressed in manual programming. High-profile examples are natural language processing, computer vision, search and recommendation systems, and, more recently, generative content systems, such as ChatGPT (text) and Stable Diffusion (text-to-image).
While the current wave of interest, investment and application is unprecedented, there are reasons to look at the latest resurgence of AI with a degree of skepticism. AI is one of the few technologies to have gone through several hype cycles since its origins as an academic discipline in 1956. These periods are often referred to as “AI summers” at the height of excitement and investment in the technology and “AI winters” during the lows.
With faster and more affordable computers, new sources of data for model training, and sensors that enable machines to better understand the physical world, new and innovative AI applications emerge. When researchers reach the technological limits of the day, the funding and interest dries out.
AI applications that prove to be useful are integrated into mainstream software and often stop being considered AI, as part of a phenomenon called “the AI effect.” In the past, this has happened to computers playing chess, optical character recognition, machine translation, email spam filters, satellite navigation systems, and personal digital assistants, such as Siri and Alexa. Applications of AI with bad product-market fit are abandoned.
Data center operators, like other managers across industry, tend to react to the hype cycles with waves of inflated or dampened expectations. In 2019, 29% of respondents to Uptime’s annual survey said they believed that AI would reduce the need for data center staff within the next five years (Figure 1). Nearly five years later, we don’t see any evidence of this reduction taking place.
Figure 1: More operators expect AI to reduce staffing requirements in the near term
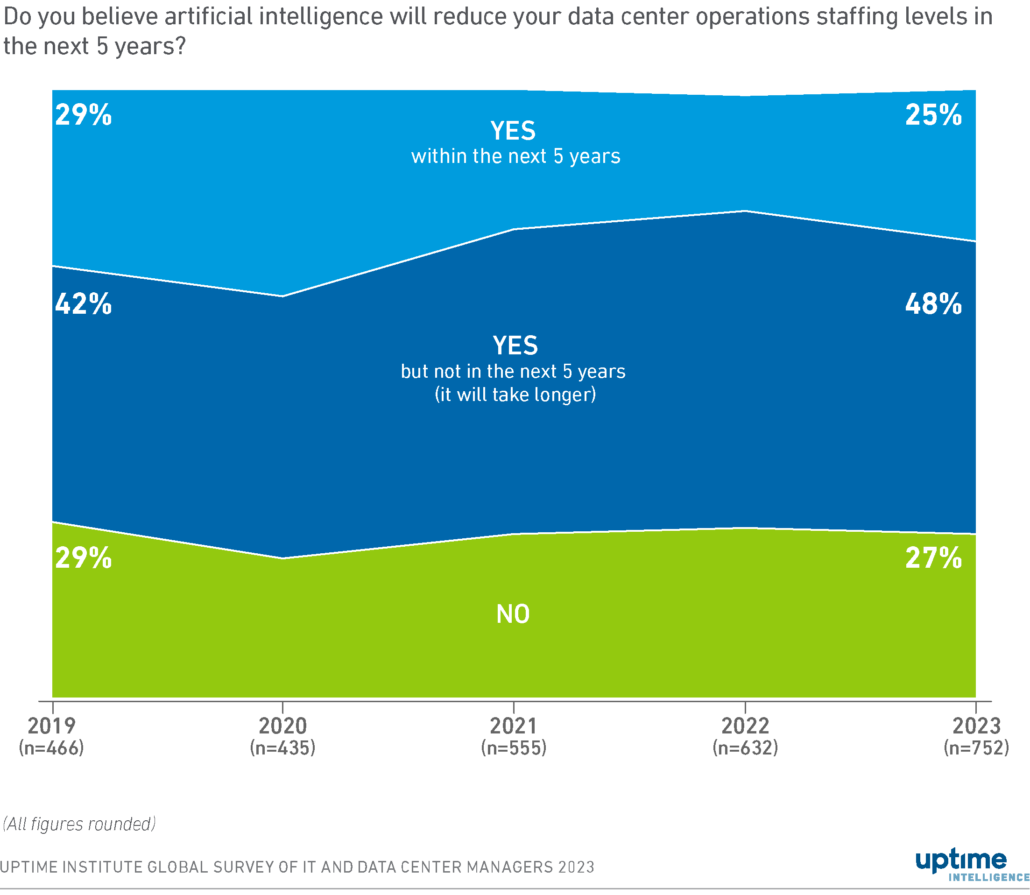
AI in the data center
Some AI-based applications have made it into the data center. AI is currently used for dynamic power and cooling optimization, in anomaly detection, predictive maintenance, and other types of predictive analytics.
AI is rarely integrated into data center management tools as a control mechanism. Instead, it is used to advise facility operators. Ceding control of the facility to algorithms or models might make the infrastructure more efficient, but it would also expose the data center to new types of risk — and arguably new single points of failure in the AI mechanism itself. Any mistakes in model design or operation could result in prolonged outages, which could cost millions of dollars. This is not a gamble that operators are currently willing to take.
Increased media coverage of AI has also created more awareness of the faults and limitations that exist within the current generation of AI-based tools, which drives further caution. One such fault that has gained prominence in 2023 is the concept of “artificial hallucinations,” which describes the tendency of generative AI models to occasionally produce confident but inaccurate responses on factual matters. Other issues include the lack of decision-making transparency and accountability (often described as the “black box” problem), and concerns over the security of the data that is provided to train the models.
Nothing is new
It is worth noting that AI has had plenty of time to make inroads into the data center: US company Vigilent — an AI-based tool developer focused on digital infrastructure — has been applying ML in its cooling equipment optimization system since 2008. Some of the vendors to integrate this technology in their data center management tools include Schneider Electric, Siemens and Hitachi Vantara.
Vigilent is not alone in offering this kind of service. Recent entries in the cooling optimization product category include Phaidra in the US (established in 2019) and Coolgradient in Europe (founded in 2021). The former was founded by some members of the DeepMind team, which built an ML model for a Google data center that reportedly cut down the power consumption of cooling equipment by 40%.
What these tools, which represent some of the most successful implementations of AI in data center operations, have in common is their intention to augment humans rather than replace them — they drive cooling systems with a level of detail that the human brain alone would find difficult, if not impossible, to achieve.
The impact on jobs
According to Data Center Career Pathfinder — the tool developed in collaboration between Uptime Institute and some of the world’s largest data center operators — there are at least 25 distinct career options in data center operations and another 25 in operations engineering. These roles include engineers, mechanics, electricians, HVAC technicians, supplier quality managers, environmental health and safety coordinators, and cleaning specialists.
Most operations jobs require a physical presence at the site and interaction with physical equipment within the data center. No matter how intelligent, a software system cannot install a server or fix an ailing generator set.
There are, however, a few data center positions that may be at immediate risk from AI tools. The need for preventative maintenance planners might be reduced since the current generation of AI-based tools can predict failure rates and suggest optimal, condition-based maintenance schedules through advanced statistical methods. There may also be less need for physical security staff: CCTV systems equipped with detection, recognition and tracking features are able to alert the front desk if someone is in the facility without the right credentials. In the future, such systems will get smarter and cover a growing number of threat types through complex pattern recognition in and around the data center.
At the same time, the data center industry is suffering from severe staffing shortages. Half of the respondents to Uptime’s annual survey said they are experiencing difficulties in finding qualified candidates for open jobs. Even if AI-based tools become reliable enough to take over some of the duties of human employees, the likely impact would be to reduce the need for additional hires, offsetting the deficit in staff recruitment, rather than replace those already employed in data centers.
On balance, AI is not going to devour many, if any, jobs in data centers. Equally, it is premature to look to AI as a short-term fix to the industry’s staffing issues. Instead, the data center industry needs to take steps to draw in more staff through advertising the benefits of working in the sector to qualified job seekers, and particularly through attracting the younger cohorts by offering a clear path for training and career progression.
Perhaps this time around AI will really change the fabric of society and the nature of work. In the meantime, developing and deploying smarter AI systems will require a great deal more infrastructure capacity, which will generate new data center jobs before the technology displaces any.
The Uptime Intelligence View
Large language models and generative AI applications are making the headlines but are unlikely to find many uses in data center management and operation. Instead, the current hype cycle might make operators more amenable to better established and understood types of AI — those that have been deployed in data centers over the past 15 years but haven’t reached mainstream adoption. There is little doubt that, eventually, some jobs will be automated out of existence through AI-based software. However, data centers will continue to provide secure employment and operational staff, in particular, will continue to be in high demand.